Distributed Learning for Kernel Mode-Based Regression
摘要:
We in this paper propose a parametric kernel mode-based regression built on the mode value, which can achieve robust and efficient estimators for datasets containing outliers or featuring heavy-tailed distributions. We show that the resultant estimators can arrive at the highest asymptotic breakdown point of 0.5. To address the challenges posed by massive datasets, we then integrate this regression method with distributed statistical learning techniques, which greatly reduces the required amount of primary memory and simultaneously accommodates heterogeneity in the estimation process. By approximating the local kernel objective function with a least squares format, we are able to preserve compact statistics for each worker machine, facilitating the reconstruction of estimates for the entire dataset with minimal asymptotic approximation error. With the help of a Gaussian kernel, we introduce an iteration algorithm based on the expectation-maximization procedure to substantially reduce the computational burden. We establish the asymptotic properties of the developed mode-based estimators, demonstrating that the suggested estimator for massive datasets is statistically as efficient as the global mode-based estimator using the full dataset. Additionally, we explore shrinkage estimation through local quadratic approximation, showcasing that the resulting estimator possesses the oracle property through an adaptive LASSO approach. The finite sample performance of the developed method is illustrated using simulations as well as real data analysis.
报告时间:2023年12月7日,下午2:00
线下地点:经济学院6-210会议室
主办单位:浙江财经大学经济学院
浙江财经大学数量经济研究中心
嘉宾简介:
王涛,加拿大维多利亚大学经济系助理教授,2022年博士毕业于美国加州大学河滨分校。其研究方向为计量经济学。论文发表于Journal of Econometrics, Statistica Sinica, Journal of the Royal Statistical Society Series A, Journal of Time Series Analysis, Oxford Bulletin of Economics and Statistics等期刊。
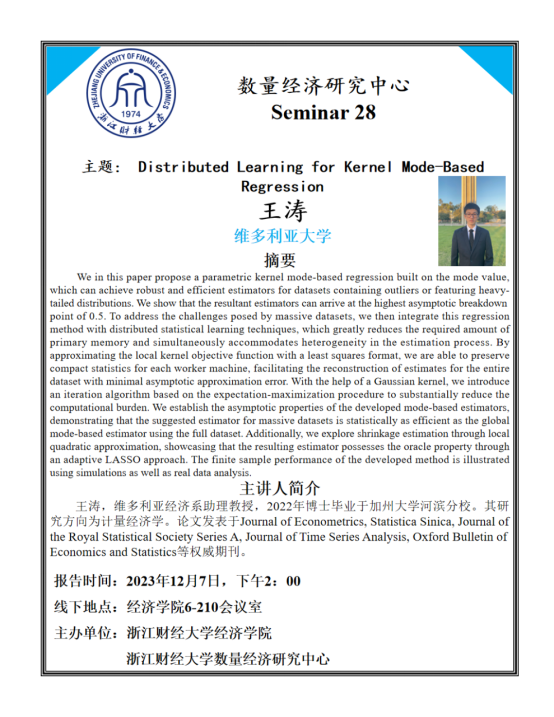